Prepare your data
This article describes data wellness concepts and actionable steps you can take to examine and optimize the readiness of your data layer before starting with Tealium Predict ML.
Data wellness concepts
This section defines basic data wellness concepts you can use to examine, audit, and optimize to improve the overall wellness of your data. The goal is to ensure that you start with a trusted data foundation. As data maturity levels differ between businesses based on a number of factors, it is important to review and consider each of the following concepts.
After reviewing and understanding the concepts, advance to the following data readiness steps:
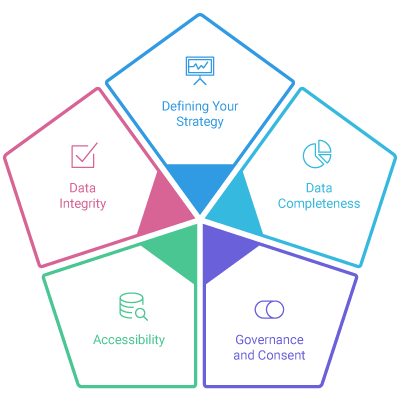
-
Strategy
Define your business goals and your strategy. Define what do want to accomplish with machine learning technology, identify your audiences, and decide what data you want to capture and act on. -
Data Completeness
Examine the volume and completeness of your data. As always, better data produces better outcomes. -
Governance and Consent
Review and understand compliance practices and requirements regarding data usage. -
Accessibility
Ensure that the insights you identify are accessible to other tools within your “tech stack”. -
Data Integrity
Review your Tealium AudienceStream CDP implementation and compare the results of your data to the of data you want to collect, capture, and act on.
To learn more about data readiness and review the wellness checklist, see the Data Readiness for Machine Learning Checklist.
Data wellness services
If you need help getting started, we offer a “Data Wellness” services package to assist in getting your data ready to use with Tealium Predict. To learn how you can benefit from this service according to your unique data, contact your account representative.
Data readiness actions
The following steps describe actions you and your teams can take to get your data foundation ready to add Tealium Predict. Use these steps as a general guideline to take the recommended actions to ensure optimal outcome from your Machine Learning models.
- Audit
Audit your data layer and AudienceStream attributes. Examine your data quality, volume, and maturity and make any adjustments as needed to improve the quality and integrity of your customer data. - Define and Integrate
Define your digital data strategy and integrate. Your data foundation and infrastructure is vendor-neutral and can be used to power your entire “tech stack” without dependencies on any one vendor. - Data Governance Practices
Review, define, and implement your data governance practices. As compliance is ever-evolving, ensure that compliance issues are revisited on a regular basis to ensure the long-term health and viability of your data. - Understand and Enrich
At this point, your event data infrastructure has not yet acquired machine learning intelligence. Enrich and organize your incoming event data to improve the quality of the insights automatically produced by AudienceStream. This step enables you to immediately activate machine learning insights across your entire implementation, regardless of whether or not other components of your implementation have machine learning capabilities. - Orchestrate
After defining your infrastructure and after your data insights are built into the way your business collects data, you can orchestrate these insights to drive action in any integrated tool of implementation. You can additionally implement your own business rules that automate data insights to flow across all your customer engagement channels in real-time
Best practices
For optimal results, we recommend training using a prediction timeframe that contains 90 or more days of data. In many circumstances, and depending on your target use case, you can successfully train models using a smaller date range and less data. As a minimum, you must train a model on at least as many days as your prediction timeframe.
This page was last updated: February 21, 2023